Enhancing Urban Resilience Against Liquefaction Using Machine Learning: A Step Towards Safer Cities
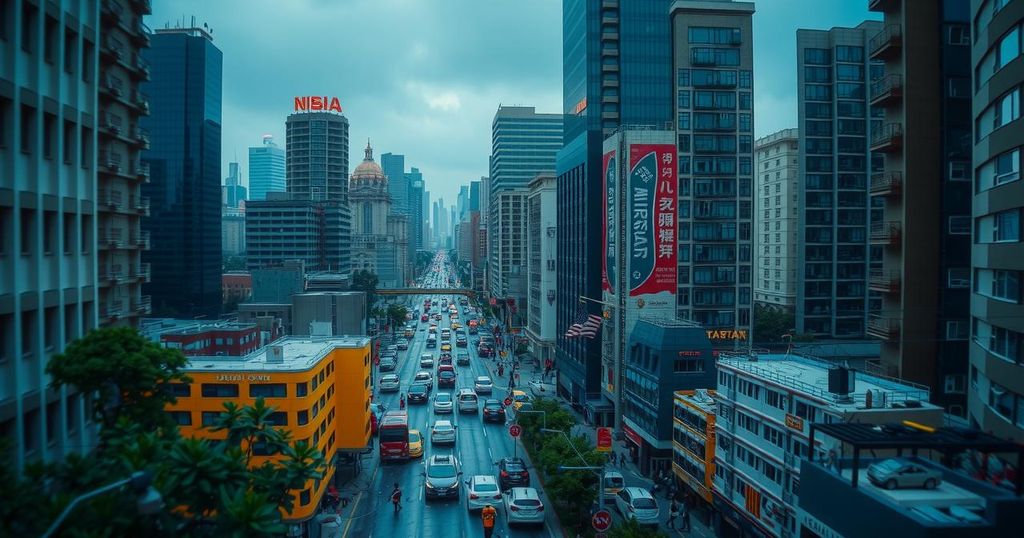
Researchers at Shibaura Institute of Technology have developed a machine learning model to improve urban resilience against liquefaction in earthquake-prone regions. Utilizing artificial neural networks to create 3D maps of soil bearing layers, they enhance city planners’ ability to identify stable construction areas and mitigate disaster risks. Historical evidence of liquefaction’s impact underscores the importance of this advancement, which aims to foster safer urban growth in Japan and beyond.
In a significant advancement towards enhancing urban resilience, researchers at Shibaura Institute of Technology have developed a machine learning model aimed at improving the safety of cities, particularly in earthquake-prone regions like Japan. This innovative model leverages artificial neural networks (ANNs) and ensemble learning techniques to produce detailed three-dimensional (3D) maps that delineate the depth of soil bearing layers, which serve as crucial indicators of soil stability during seismic events. By analyzing geological data from 433 locations in Setagaya, Tokyo, the researchers have successfully created contour maps that illuminate areas susceptible to liquefaction, thereby aiding city planners and disaster management authorities in their efforts to identify suitable construction sites and enhance disaster preparedness strategies. Liquefaction poses a critical threat to urban infrastructure during earthquakes, wherein saturated, loose soils can lose their strength and behave like a liquid, leading to severe structural damages and disruptions in essential services. Historical references to liquefaction damage underscore its significance; for instance, the 2011 Tōhoku earthquake resulted in widespread liquefaction that devastated approximately 1,000 homes and significantly impacted water and sewage systems in Christchurch during its 2011 earthquake. In their recent study published in “Smart Cities,” the authors, Professor Shinya Inazumi and student Yuxin Cong, have successfully employed machine learning to create predictive models that can estimate soil behavior with remarkable precision. Traditional soil testing approaches have limitations in coverage, often failing to provide a comprehensive understanding of soil stability across a city. In contrast, their model allows for a broader assessment of intricate underground structures, offering valuable insights for urban development. The research team meticulously gathered and analyzed data regarding bearing layer depth using standard penetration tests and mini-ram sounding tests. Their innovative use of bagging—training the model on various subsets of data—has led to a notable enhancement in predictive accuracy by 20%. The resultant maps serve as indispensable resources for civil engineers and disaster risk assessors in their endeavors to identify both safe construction zones and areas requiring heightened vigilance to mitigate liquefaction risks. This groundbreaking work not only exemplifies the pivotal role of machine learning in geotechnical engineering but also advocates for adopting data-driven strategies to anticipate urban challenges. Professor Inazumi expresses optimism regarding the future, stating that integrating advanced AI models into urban planning will significantly mitigate liquefaction risks, thus fostering the development of safer and smarter cities. Looking ahead, the researchers aim to refine their models further by incorporating additional geological variables and designing tailored models for coastal and non-coastal regions to enhance predictive capabilities related to groundwater influence, a critical element in liquefaction scenarios.
The use of machine learning in understanding and predicting soil stability is of paramount importance in earthquake-stricken regions, where natural disasters can wreak havoc on urban infrastructure. Liquefaction, a phenomenon typically triggered by intense seismic activity, highlights the necessity for innovative approaches in urban planning and disaster management. As cities continue to expand, integrating advanced technology into safety assessments becomes essential to mitigate risks associated with natural disasters, particularly in areas like Japan, which face the constant threat posed by earthquakes. The convergence of artificial intelligence and geotechnical engineering provides a robust framework to enhance urban resilience through data-informed strategies, making construction practices safer and more efficient.
In conclusion, the research conducted by Professor Shinya Inazumi and Yuxin Cong represents a substantial contribution to the field of urban safety by harnessing machine learning to predict soil behavior during earthquakes. This model not only highlights the vulnerability of urban areas to liquefaction but also facilitates informed decision-making regarding construction and infrastructure planning. As cities contend with the increasing challenges of natural disasters, such innovative approaches are vital for fostering resilience and ensuring the safety of urban populations in seismically active regions. The future of urban planning must embrace these advancements to effectively protect against the threats posed by liquefaction and other natural phenomena.
Original Source: www.preventionweb.net